AI is no longer just an innovation initiative—it’s on track to become one of the biggest line items in enterprise IT. With generative AI projected to grow from $40 billion in 2022 to $1.3 trillion by 2032, managing AI spend is moving to the top of the agenda.
To keep pace, companies are adopting multi-model, multi-provider strategies—mirroring the rise of multicloud—to ensure flexibility, resilience, and control in a fast-changing ecosystem. This shift makes FinOps for AI a must-have, not a nice-to-have.
This article isn’t about how AI can enhance FinOps—it’s about why FinOps is now essential for managing the financial reality of enterprise AI.
Why AI need FinOps? Spending will be massive
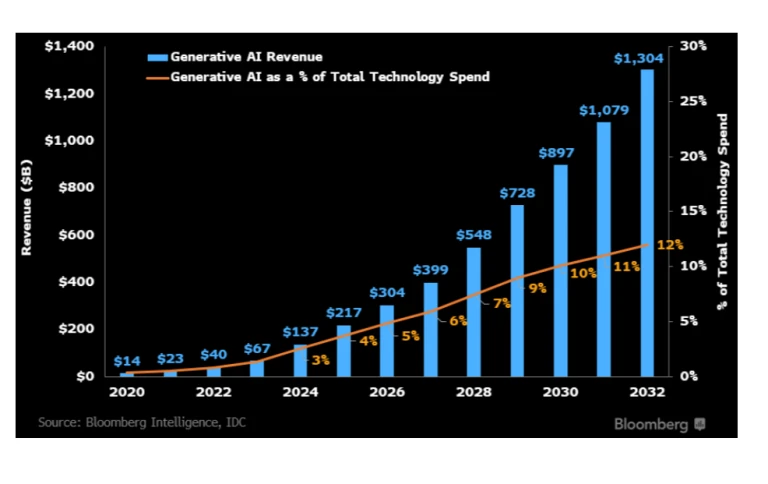
Understanding the financial implications of AI adoption starts with grasping the scale of the market opportunity. According to Bloomberg Intelligence, the generative AI industry is projected to grow from $40 billion in 2022 to $1.3 trillion by 2032, reflecting a compound annual growth rate (CAGR) of 42%. This growth trajectory is among the fastest ever seen in the software industry.
Bloomberg’s analysis highlights several key drivers behind this surge:
- $280 billion in new software revenue will be created by generative AI, powered by copilots, digital assistants, and AI-native infrastructure tools.
- The biggest beneficiaries are expected to be cloud and AI infrastructure providers such as Amazon Web Services, Microsoft, Google, and Nvidia, as enterprises accelerate their shift to the cloud to support training and inference workloads.
- By 2032, generative AI could represent 10% of total global IT, software, advertising, and gaming spend, up from less than 1% today.
Understanding AI Cost Structures
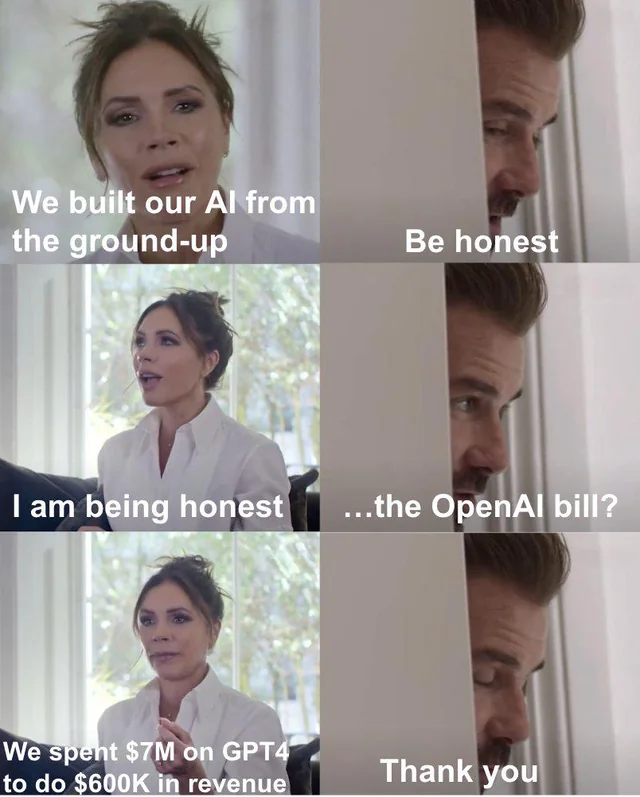
Unlike traditional software with fixed licensing fees, generative AI services typically use consumption-based pricing models centered around “tokens“—the fundamental units that AI models process:
Token-based pricing: Providers charge based on the number of tokens processed, with separate rates for input tokens (prompts sent to the model) and output tokens (responses generated). A token roughly represents 4 characters in English text.
Model tier differentials: More capable models command premium prices. For example, GPT-4 might cost 10-20 times more per token than simpler models like GPT-3.5.
Batch processing economics: Some providers offer lower prices for batch jobs (Up to 50% discount)—processing large volumes of prompts in a non-interactive, deferred mode—versus real-time API calls. This can significantly reduce costs for workloads that don’t require immediate responses.
Volume discounts: Enterprise customers can often negotiate volume-based discounts that reduce per-token costs as usage scales.
Additional service fees: Beyond core model access, providers often charge for related services such as vector embeddings, fine-tuning, or specialized model deployments.
Latency vs. cost tradeoffs: Faster response times often come at premium prices, creating an economic incentive to optimize between performance and cost.
This complex pricing landscape means that similar AI workloads can have dramatically different costs depending on model selection, prompt engineering efficiency, and implementation details.
Companies already use multiple AI providers
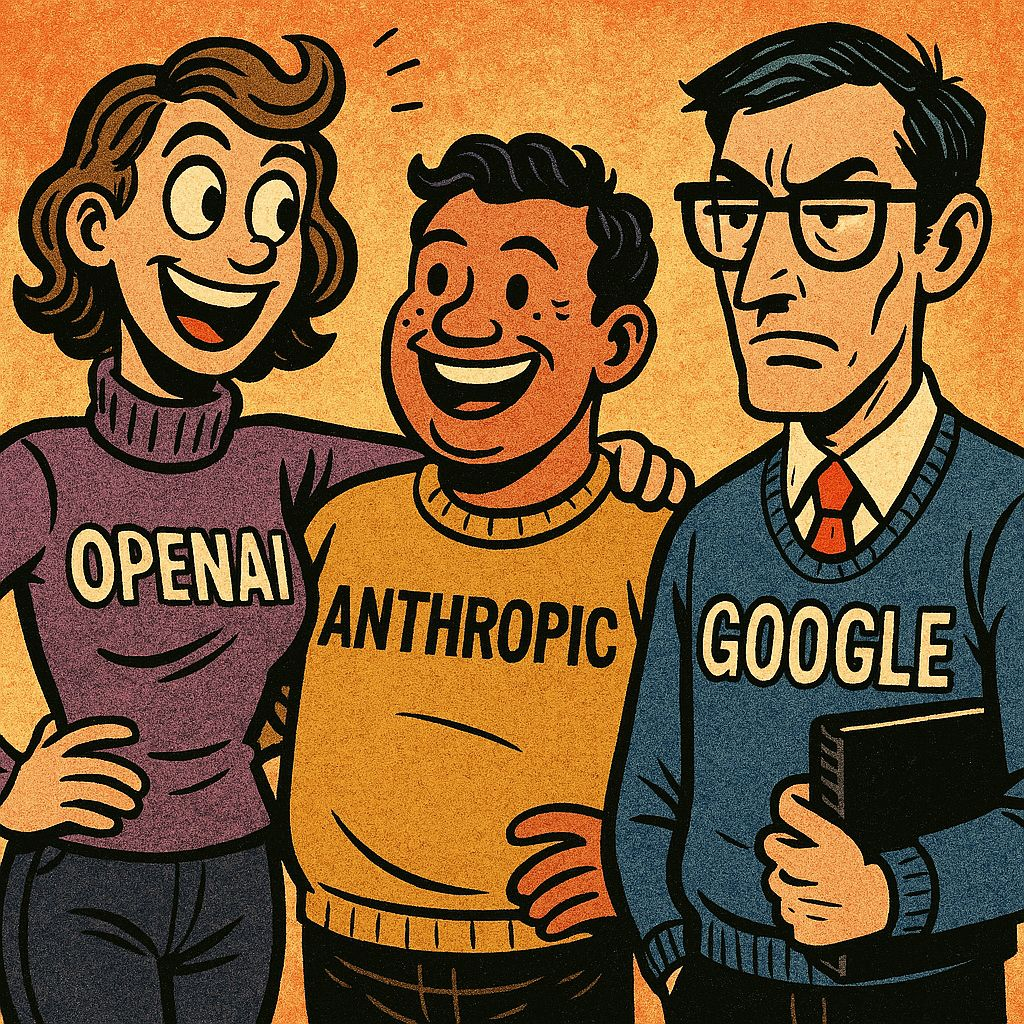
Just as organizations embraced multicloud to avoid vendor lock-in and access best-of-breed services, they’re now implementing similar strategies with generative AI. Each AI model offers distinct capabilities, pricing structures, and performance characteristics that make them suitable for different use cases.
Companies are discovering that no single AI model excels at everything. Some models demonstrate superior reasoning capabilities, while others excel at code generation, creative writing, or multilingual tasks. By leveraging multiple models, businesses can match each task to the most suitable AI, optimizing for both performance and cost.
To see what model is best for what and at which cost there is a great website that compares and benchmarks all AI models: https://lmarena.ai/
Strategic Benefits of a Multi-Model Approach for AI
Several factors are driving this diversification:
Specialized capabilities: Different models excel in different domains. While one might generate superior code, another might excel at summarizing complex documents or creating amazing images. At the current level of development no all generative AI providers offer all services. OpenAI has the widest coverage by covering almost all use cases.
Cost optimization: Usage-based pricing varies significantly between providers and models. Some tasks may be more economically performed by lighter, less expensive models while reserving premium models for complex tasks requiring advanced reasoning.
Risk mitigation: Relying on a single AI provider creates significant business continuity risks. Service disruptions, pricing changes, or policy shifts from a sole provider could severely impact operations.
Compliance requirements: Data residency, privacy regulations, and industry-specific compliance needs often necessitate using region-specific or specially certified AI providers.
When you’re running several AI models, keeping tabs on costs gets messy fast. That’s why a central platform for visibility and control is essential.
The Growing Importance of AI Cost Management
As AI usage proliferates across organizations, managing the associated costs is becoming increasingly critical. The consumption-based pricing models of AI services, coupled with the emerging trend of multi-model strategies mirroring the complexity of multicloud environments, create a financial landscape that closely resembles cloud computing—unpredictable, potentially escalating, and requiring proactive management, thus highlighting the essential need for robust cloud cost management solutions adapted for the AI domain.
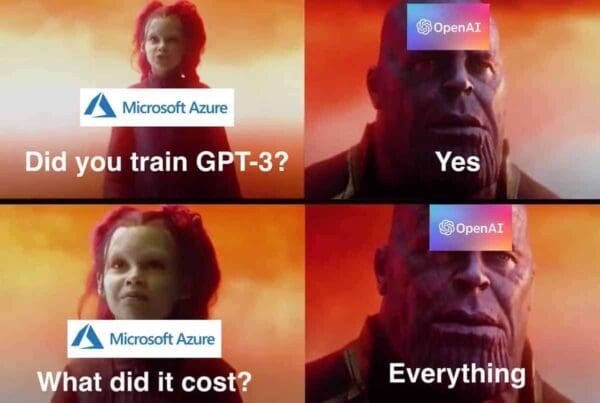
FinOps for AI: An Emerging Necessity
The established discipline of FinOps (Financial Operations) is naturally extending to encompass AI expenditures. Just as organizations needed visibility and control over their cloud spending, they now require similar capabilities for their AI investments.
Key components of an AI FinOps approach include
- Consolidated spending data across multiple AI providers to gain comprehensive visibility into total AI expenditure.
- Tracking AI costs and revenue by department, project, application, or business outcome drives accountability and identifies optimization opportunities.
- Analyzing cost-performance tradeoffs helps direct workloads to the most economically efficient models based on specific requirements.
- Optimizing prompts to reduce token consumption while maintaining quality outputs can significantly reduce costs, while predicting future AI spending based on current trends and planned initiatives supports budgeting processes.
- Many organizations are also implementing intelligent caching of AI responses for repetitive queries to minimize redundant API calls.
Integration of AI spend with Existing FinOps Practices
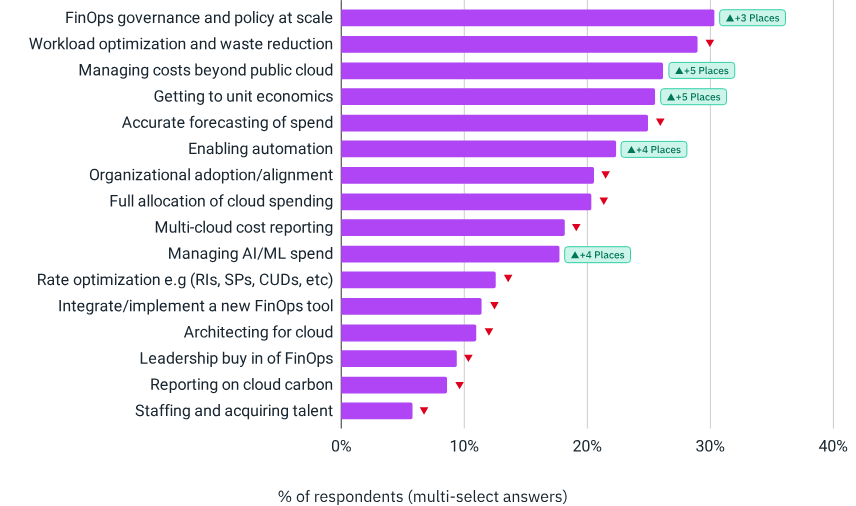
For many organizations, AI costs represent a natural extension of existing cloud and SaaS cost management initiatives. The most effective approach integrates AI spending into a unified FinOps framework that encompasses all technology investments. This holistic view enables organizations to make informed decisions about resource allocation across their entire technology portfolio, optimizing spending based on business value rather than treating each category in isolation.
According to the FinOps Foundation’s 2025 “State of FinOps” report, FinOps teams are moving toward platform-wide visibility—combining infrastructure, SaaS, AI, Licenses, Private cloud and data center into a single cost governance model. In this context, AI is not a special case; it’s another high-impact domain that must be tracked, allocated, and optimized using FinOps best practices like real-time monitoring, unit cost metrics, and collaboration between finance, engineering, and product teams.
Technical Approaches to AI Cost Optimization
Several technical strategies can help organizations optimize their AI expenditures. Implementing intelligent routing systems can direct requests to the most cost-effective model capable of handling a particular task. Organizations should evaluate when it’s more economical to fine-tune a smaller model versus using a larger pre-trained model for specific use cases. Grouping similar requests takes advantage of bulk processing discounts offered by some providers. Storing and reusing vector embeddings rather than regenerating them for each similar query saves costs, as does setting appropriate maximum token limits to prevent runaway costs from unexpectedly verbose outputs.
In this article, I give concrete tips on how to optimize cost for OpenAI.
Looking Ahead: The Future of FinOps for AI
Generative AI is no longer an experimental playground for innovation teams—it’s becoming a core layer of enterprise operations, embedded in products, workflows, and customer experiences. As its strategic importance grows, so does the urgency to bring financial discipline to AI deployments.
The next frontier of FinOps is here. AI workloads—dynamic, expensive, and complex—require the same rigor, tooling, and cross-functional collaboration that transformed cloud cost management in the last decade. Organizations that treat AI cost optimization as a first-class discipline will not only avoid budget surprises—they’ll gain a competitive edge in performance, speed, and scalability.
FinOps for AI isn’t just about controlling costs. It’s about enabling smart growth, aligning spend with value, and empowering teams to innovate without financial blind spots.
In a market racing toward $1.3 trillion, the winners will be those who can harness the full power of AI—efficiently, transparently, and strategically.
At Holori we will start implementing cost visibility and alerts for majors AI providers in 2025 so stay tuned! In the meantime you can already track and optimize your cloud costs: https://app.holori.com/
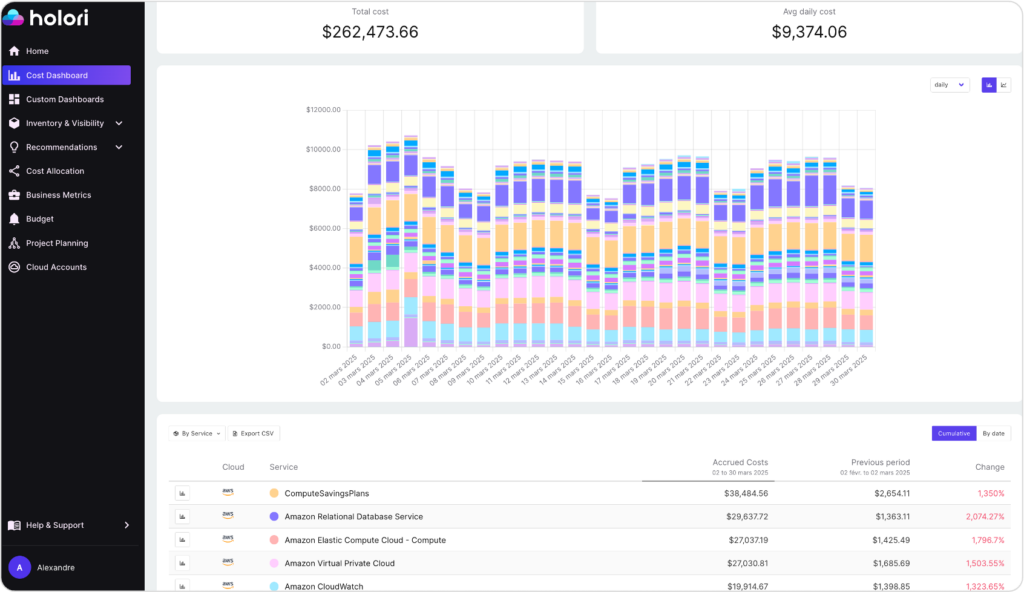